Using OCP to enumerate adsorbates on alloy catalyst surfaces#
In the previous example, we constructed slab models of adsorbates on desired sites. Here we leverage code to automate this process. The goal in this section is to generate candidate structures, compute energetics, and then filter out the most relevant ones.
from fairchem.core.common.relaxation.ase_utils import OCPCalculator
import ase.io
from ase.optimize import BFGS
import sys
from scipy.stats import linregress
import pickle
import matplotlib.pyplot as plt
import time
from fairchem.data.oc.core import Adsorbate, AdsorbateSlabConfig, Bulk, Slab
import os
from glob import glob
import pandas as pd
from fairchem.data.oc.utils import DetectTrajAnomaly
# Set random seed to ensure adsorbate enumeration yields a valid candidate
# If using a larger number of random samples this wouldn't be necessary
import numpy as np
np.random.seed(22)
from fairchem.core.models.model_registry import model_name_to_local_file
checkpoint_path = model_name_to_local_file('EquiformerV2-31M-S2EF-OC20-All+MD', local_cache='/tmp/fairchem_checkpoints/')
checkpoint_path
'/tmp/fairchem_checkpoints/eq2_31M_ec4_allmd.pt'
Introduction#
We will reproduce Fig 6b from the following paper: Zhou, Jing, et al. “Enhanced Catalytic Activity of Bimetallic Ordered Catalysts for Nitrogen Reduction Reaction by Perturbation of Scaling Relations.” ACS Catalysis 134 (2023): 2190-2201 (https://doi.org/10.1021/acscatal.2c05877).
The gist of this figure is a correlation between H* and NNH* adsorbates across many different alloy surfaces. Then, they identify a dividing line between these that separates surfaces known for HER and those known for NRR.
To do this, we will enumerate adsorbate-slab configurations and run ML relaxations on them to find the lowest energy configuration. We will assess parity between the model predicted values and those reported in the paper. Finally we will make the figure and assess separability of the NRR favored and HER favored domains.
Enumerate the adsorbate-slab configurations to run relaxations on#
Be sure to set the path in fairchem/data/oc/configs/paths.py
to point to the correct place or pass the paths as an argument. The database pickles can be found in fairchem/data/oc/databases/pkls
(some pkl files are only downloaded by running the command python src/fairchem/core/scripts/download_large_files.py oc
from the root of the fairchem repo). We will show one explicitly here as an example and then run all of them in an automated fashion for brevity.
import fairchem.data.oc
from pathlib import Path
db = Path(fairchem.data.oc.__file__).parent / Path('databases/pkls/adsorbates.pkl')
db
PosixPath('/home/runner/work/fairchem/fairchem/src/fairchem/data/oc/databases/pkls/adsorbates.pkl')
Work out a single example#
We load one bulk id, create a bulk reference structure from it, then generate the surfaces we want to compute.
bulk_src_id = "oqmd-343039"
adsorbate_smiles_nnh = "*N*NH"
adsorbate_smiles_h = "*H"
bulk = Bulk(bulk_src_id_from_db = bulk_src_id, bulk_db_path="NRR_example_bulks.pkl")
adsorbate_H = Adsorbate(adsorbate_smiles_from_db = adsorbate_smiles_h, adsorbate_db_path=db)
adsorbate_NNH = Adsorbate(adsorbate_smiles_from_db = adsorbate_smiles_nnh, adsorbate_db_path=db)
slab = Slab.from_bulk_get_specific_millers(bulk= bulk, specific_millers = (1, 1, 1))
slab
[Slab: (Ag36Pd12, (1, 1, 1), 0.16666666666666669, True)]
We now need to generate potential placements. We use two kinds of guesses, a heuristic and a random approach. This cell generates 13 potential adsorption geometries.
# Perform heuristic placements
heuristic_adslabs = AdsorbateSlabConfig(slab[0], adsorbate_H, mode="heuristic")
# Perform random placements
# (for AdsorbML we use `num_sites = 100` but we will use 4 for brevity here)
random_adslabs = AdsorbateSlabConfig(slab[0], adsorbate_H, mode="random_site_heuristic_placement", num_sites=4)
adslabs = [*heuristic_adslabs.atoms_list, *random_adslabs.atoms_list]
len(adslabs)
13
Let’s see what we are looking at. It is a little tricky to see the tiny H atom in these figures, but with some inspection you can see there are ontop, bridge, and hollow sites in different places. This is not an exhaustive search; you can increase the number of random placements to check more possibilities. The main idea here is to increase the probability you find the most relevant sites.
import matplotlib.pyplot as plt
from ase.visualize.plot import plot_atoms
fig, axs = plt.subplots(4, 4)
for i, slab in enumerate(adslabs):
plot_atoms(slab, axs[i % 4, i // 4]);
axs[i % 4, i // 4].set_axis_off()
for i in range(16):
axs[i % 4, i // 4].set_axis_off()
plt.tight_layout()
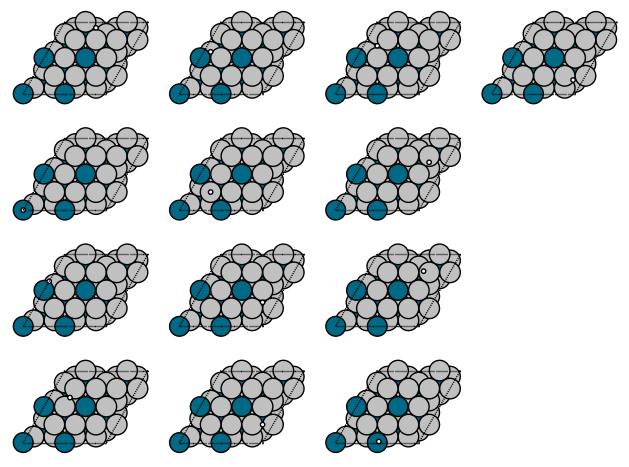
Run an ML relaxation#
We will use an ASE compatible calculator to run these.
Running the model with BFGS prints at each relaxation step which is a lot to print. So we will just run one to demonstrate what happens on each iteration.
os.makedirs(f"data/{bulk_src_id}_{adsorbate_smiles_h}", exist_ok=True)
# Define the calculator
calc = OCPCalculator(checkpoint_path=checkpoint_path, cpu=False) # if you have a GPU
# calc = OCPCalculator(checkpoint_path=checkpoint_path, cpu=True) # If you have CPU only
/home/runner/work/fairchem/fairchem/src/fairchem/core/models/scn/spherical_harmonics.py:23: FutureWarning: You are using `torch.load` with `weights_only=False` (the current default value), which uses the default pickle module implicitly. It is possible to construct malicious pickle data which will execute arbitrary code during unpickling (See https://github.com/pytorch/pytorch/blob/main/SECURITY.md#untrusted-models for more details). In a future release, the default value for `weights_only` will be flipped to `True`. This limits the functions that could be executed during unpickling. Arbitrary objects will no longer be allowed to be loaded via this mode unless they are explicitly allowlisted by the user via `torch.serialization.add_safe_globals`. We recommend you start setting `weights_only=True` for any use case where you don't have full control of the loaded file. Please open an issue on GitHub for any issues related to this experimental feature.
_Jd = torch.load(os.path.join(os.path.dirname(__file__), "Jd.pt"))
/home/runner/work/fairchem/fairchem/src/fairchem/core/models/equiformer_v2/wigner.py:10: FutureWarning: You are using `torch.load` with `weights_only=False` (the current default value), which uses the default pickle module implicitly. It is possible to construct malicious pickle data which will execute arbitrary code during unpickling (See https://github.com/pytorch/pytorch/blob/main/SECURITY.md#untrusted-models for more details). In a future release, the default value for `weights_only` will be flipped to `True`. This limits the functions that could be executed during unpickling. Arbitrary objects will no longer be allowed to be loaded via this mode unless they are explicitly allowlisted by the user via `torch.serialization.add_safe_globals`. We recommend you start setting `weights_only=True` for any use case where you don't have full control of the loaded file. Please open an issue on GitHub for any issues related to this experimental feature.
_Jd = torch.load(os.path.join(os.path.dirname(__file__), "Jd.pt"))
/home/runner/work/fairchem/fairchem/src/fairchem/core/models/escn/so3.py:23: FutureWarning: You are using `torch.load` with `weights_only=False` (the current default value), which uses the default pickle module implicitly. It is possible to construct malicious pickle data which will execute arbitrary code during unpickling (See https://github.com/pytorch/pytorch/blob/main/SECURITY.md#untrusted-models for more details). In a future release, the default value for `weights_only` will be flipped to `True`. This limits the functions that could be executed during unpickling. Arbitrary objects will no longer be allowed to be loaded via this mode unless they are explicitly allowlisted by the user via `torch.serialization.add_safe_globals`. We recommend you start setting `weights_only=True` for any use case where you don't have full control of the loaded file. Please open an issue on GitHub for any issues related to this experimental feature.
_Jd = torch.load(os.path.join(os.path.dirname(__file__), "Jd.pt"))
/home/runner/work/fairchem/fairchem/src/fairchem/core/common/relaxation/ase_utils.py:200: FutureWarning: You are using `torch.load` with `weights_only=False` (the current default value), which uses the default pickle module implicitly. It is possible to construct malicious pickle data which will execute arbitrary code during unpickling (See https://github.com/pytorch/pytorch/blob/main/SECURITY.md#untrusted-models for more details). In a future release, the default value for `weights_only` will be flipped to `True`. This limits the functions that could be executed during unpickling. Arbitrary objects will no longer be allowed to be loaded via this mode unless they are explicitly allowlisted by the user via `torch.serialization.add_safe_globals`. We recommend you start setting `weights_only=True` for any use case where you don't have full control of the loaded file. Please open an issue on GitHub for any issues related to this experimental feature.
checkpoint = torch.load(checkpoint_path, map_location=torch.device("cpu"))
WARNING:root:Detected old config, converting to new format. Consider updating to avoid potential incompatibilities.
INFO:root:amp: true
cmd:
checkpoint_dir: /home/runner/work/fairchem/fairchem/docs/tutorials/NRR/checkpoints/2025-03-29-04-58-40
commit: core:b88b66e,experimental:NA
identifier: ''
logs_dir: /home/runner/work/fairchem/fairchem/docs/tutorials/NRR/logs/wandb/2025-03-29-04-58-40
print_every: 100
results_dir: /home/runner/work/fairchem/fairchem/docs/tutorials/NRR/results/2025-03-29-04-58-40
seed: null
timestamp_id: 2025-03-29-04-58-40
version: 0.1.dev1+gb88b66e
dataset:
format: trajectory_lmdb_v2
grad_target_mean: 0.0
grad_target_std: 2.887317180633545
key_mapping:
force: forces
y: energy
normalize_labels: true
target_mean: -0.7554450631141663
target_std: 2.887317180633545
transforms:
normalizer:
energy:
mean: -0.7554450631141663
stdev: 2.887317180633545
forces:
mean: 0.0
stdev: 2.887317180633545
evaluation_metrics:
metrics:
energy:
- mae
forces:
- forcesx_mae
- forcesy_mae
- forcesz_mae
- mae
- cosine_similarity
- magnitude_error
misc:
- energy_forces_within_threshold
primary_metric: forces_mae
gp_gpus: null
gpus: 0
logger: wandb
loss_functions:
- energy:
coefficient: 4
fn: mae
- forces:
coefficient: 100
fn: l2mae
model:
alpha_drop: 0.1
attn_activation: silu
attn_alpha_channels: 64
attn_hidden_channels: 64
attn_value_channels: 16
distance_function: gaussian
drop_path_rate: 0.1
edge_channels: 128
ffn_activation: silu
ffn_hidden_channels: 128
grid_resolution: 18
lmax_list:
- 4
max_neighbors: 20
max_num_elements: 90
max_radius: 12.0
mmax_list:
- 2
name: equiformer_v2
norm_type: layer_norm_sh
num_distance_basis: 512
num_heads: 8
num_layers: 8
num_sphere_samples: 128
otf_graph: true
proj_drop: 0.0
regress_forces: true
sphere_channels: 128
use_atom_edge_embedding: true
use_gate_act: false
use_grid_mlp: true
use_pbc: true
use_s2_act_attn: false
weight_init: uniform
optim:
batch_size: 8
clip_grad_norm: 100
ema_decay: 0.999
energy_coefficient: 4
eval_batch_size: 8
eval_every: 10000
force_coefficient: 100
grad_accumulation_steps: 1
load_balancing: atoms
loss_energy: mae
loss_force: l2mae
lr_initial: 0.0004
max_epochs: 3
num_workers: 8
optimizer: AdamW
optimizer_params:
weight_decay: 0.001
scheduler: LambdaLR
scheduler_params:
epochs: 1009275
lambda_type: cosine
lr: 0.0004
lr_min_factor: 0.01
warmup_epochs: 3364.25
warmup_factor: 0.2
outputs:
energy:
level: system
forces:
eval_on_free_atoms: true
level: atom
train_on_free_atoms: true
relax_dataset: {}
slurm:
additional_parameters:
constraint: volta32gb
cpus_per_task: 9
folder: /checkpoint/abhshkdz/open-catalyst-project/logs/equiformer_v2/8307793
gpus_per_node: 8
job_id: '8307793'
job_name: eq2s_051701_allmd
mem: 480GB
nodes: 8
ntasks_per_node: 8
partition: learnaccel
time: 4320
task:
dataset: trajectory_lmdb_v2
eval_on_free_atoms: true
grad_input: atomic forces
labels:
- potential energy
primary_metric: forces_mae
train_on_free_atoms: true
test_dataset: {}
trainer: ocp
val_dataset: {}
INFO:root:Loading model: equiformer_v2
WARNING:root:equiformer_v2 (EquiformerV2) class is deprecated in favor of equiformer_v2_backbone_and_heads (EquiformerV2BackboneAndHeads)
INFO:root:Loaded EquiformerV2 with 31058690 parameters.
INFO:root:Loading checkpoint in inference-only mode, not loading keys associated with trainer state!
/home/runner/work/fairchem/fairchem/src/fairchem/core/modules/normalization/normalizer.py:69: UserWarning: To copy construct from a tensor, it is recommended to use sourceTensor.clone().detach() or sourceTensor.clone().detach().requires_grad_(True), rather than torch.tensor(sourceTensor).
"mean": torch.tensor(state_dict["mean"]),
WARNING:root:No seed has been set in modelcheckpoint or OCPCalculator! Results may not be reproducible on re-run
Now we setup and run the relaxation.
t0 = time.time()
os.makedirs(f"data/{bulk_src_id}_H", exist_ok=True)
adslab = adslabs[0]
adslab.calc = calc
opt = BFGS(adslab, trajectory=f"data/{bulk_src_id}_H/test.traj")
opt.run(fmax=0.05, steps=100)
print(f'Elapsed time {time.time() - t0:1.1f} seconds')
Step Time Energy fmax
BFGS: 0 04:57:50 0.193125 0.716220
BFGS: 1 04:57:52 0.175033 0.673152
BFGS: 2 04:57:53 0.059609 0.388686
BFGS: 3 04:57:55 0.056406 0.223200
BFGS: 4 04:57:56 0.052641 0.171636
BFGS: 5 04:57:57 0.043569 0.134708
BFGS: 6 04:57:59 0.041664 0.118776
BFGS: 7 04:58:00 0.041299 0.080801
BFGS: 8 04:58:02 0.040331 0.065338
BFGS: 9 04:58:03 0.034359 0.081548
BFGS: 10 04:58:05 0.031854 0.078413
BFGS: 11 04:58:06 0.029978 0.070751
BFGS: 12 04:58:08 0.027736 0.059892
BFGS: 13 04:58:09 0.024113 0.037792
Elapsed time 20.3 seconds
With a GPU this runs pretty quickly. It is much slower on a CPU.
Run all the systems#
In principle you can run all the systems now. It takes about an hour though, and we leave that for a later exercise if you want. For now we will run the first two, and for later analysis we provide a results file of all the runs. Let’s read in our reference file and take a look at what is in it.
with open("NRR_example_bulks.pkl", "rb") as f:
bulks = pickle.load(f)
bulks
[{'atoms': Atoms(symbols='CuPd3', pbc=True, cell=[3.91276645, 3.91276645, 3.91276645], calculator=SinglePointDFTCalculator(...)),
'src_id': 'oqmd-349719'},
{'atoms': Atoms(symbols='Pd3Ag', pbc=True, cell=[4.02885979, 4.02885979, 4.02885979], calculator=SinglePointDFTCalculator(...)),
'src_id': 'oqmd-345911'},
{'atoms': Atoms(symbols='ScPd3', pbc=True, cell=[4.04684963, 4.04684963, 4.04684963], initial_charges=..., initial_magmoms=..., momenta=..., tags=..., calculator=SinglePointCalculator(...)),
'src_id': 'mp-2677'},
{'atoms': Atoms(symbols='Mo3Pd', pbc=True, cell=[3.96898192, 3.96898192, 3.96898192], initial_charges=..., initial_magmoms=..., momenta=..., tags=..., calculator=SinglePointCalculator(...)),
'src_id': 'mp-1186014'},
{'atoms': Atoms(symbols='Ag3Pd', pbc=True, cell=[4.14093081, 4.14093081, 4.14093081], calculator=SinglePointCalculator(...)),
'src_id': 'oqmd-343039'},
{'src_id': 'oqmd-348629',
'atoms': Atoms(symbols='Ag3Cu', pbc=True, cell=[4.09439099, 4.09439099, 4.09439099], calculator=SinglePointDFTCalculator(...))},
{'src_id': 'oqmd-343006',
'atoms': Atoms(symbols='Ag3Mo', pbc=True, cell=[4.1665424, 4.1665424, 4.1665424], calculator=SinglePointDFTCalculator(...))},
{'src_id': 'oqmd-349813',
'atoms': Atoms(symbols='AgCu3', pbc=True, cell=[3.82618693, 3.82618693, 3.82618693], calculator=SinglePointDFTCalculator(...))},
{'src_id': 'oqmd-347528',
'atoms': Atoms(symbols='Cu3Ru', pbc=True, cell=[3.72399424, 3.72399424, 3.72399424], calculator=SinglePointDFTCalculator(...))},
{'src_id': 'oqmd-344251',
'atoms': Atoms(symbols='PdTa3', pbc=True, cell=[4.13568646, 4.13568646, 4.13568646], calculator=SinglePointDFTCalculator(...))},
{'src_id': 'oqmd-343394',
'atoms': Atoms(symbols='AgMo3', pbc=True, cell=[4.00594441, 4.00594441, 4.00594441], calculator=SinglePointDFTCalculator(...))},
{'src_id': 'oqmd-344635',
'atoms': Atoms(symbols='Mo3Ru', pbc=True, cell=[3.95617571, 3.95617571, 3.95617571], calculator=SinglePointDFTCalculator(...))},
{'src_id': 'oqmd-344237',
'atoms': Atoms(symbols='MoPd3', pbc=True, cell=[3.96059535, 3.96059535, 3.96059535], calculator=SinglePointDFTCalculator(...))},
{'src_id': 'oqmd-346818',
'atoms': Atoms(symbols='Pd3Ru', pbc=True, cell=[3.93112559, 3.93112559, 3.93112559], calculator=SinglePointDFTCalculator(...))},
{'src_id': 'oqmd-349496',
'atoms': Atoms(symbols='Pd3Ta', pbc=True, cell=[3.9907085, 3.9907085, 3.9907085], calculator=SinglePointDFTCalculator(...))},
{'src_id': 'oqmd-343615',
'atoms': Atoms(symbols='MoRu3', pbc=True, cell=[3.85915122, 3.85915122, 3.85915122], calculator=SinglePointDFTCalculator(...))},
{'src_id': 'oqmd-348366',
'atoms': Atoms(symbols='AgTa3', pbc=True, cell=[4.1730103, 4.1730103, 4.1730103], calculator=SinglePointDFTCalculator(...))},
{'src_id': 'oqmd-345352',
'atoms': Atoms(symbols='AuHf3', pbc=True, cell=[4.36653536, 4.36653536, 4.36653536], calculator=SinglePointDFTCalculator(...))},
{'src_id': 'oqmd-346653',
'atoms': Atoms(symbols='AgHf3', pbc=True, cell=[4.39618436, 4.39618436, 4.39618436], calculator=SinglePointDFTCalculator(...))}]
We have 19 bulk materials we will consider. Next we extract the src-id
for each one.
bulk_ids = [row['src_id'] for row in bulks]
In theory you would run all of these, but it takes about an hour with a GPU. We provide the relaxation logs and trajectories in the repo for the next step.
These steps are embarrassingly parallel, and can be launched that way to speed things up. The only thing you need to watch is that you don’t exceed the available RAM, which will cause the Jupyter kernel to crash.
The goal here is to relax each candidate adsorption geometry and save the results in a trajectory file we will analyze later. Each trajectory file will have the geometry and final energy of the relaxed structure.
It is somewhat time consuming to run this, so in this cell we only run one example, and just the first 4 configurations for each adsorbate.
import time
from tqdm import tqdm
tinit = time.time()
# Note we're just doing the first bulk_id!
for bulk_src_id in tqdm(bulk_ids[:1]):
# Enumerate slabs and establish adsorbates
bulk = Bulk(bulk_src_id_from_db=bulk_src_id, bulk_db_path="NRR_example_bulks.pkl")
slab = Slab.from_bulk_get_specific_millers(bulk= bulk, specific_millers=(1, 1, 1))
# Perform heuristic placements
heuristic_adslabs_H = AdsorbateSlabConfig(slab[0], adsorbate_H, mode="heuristic")
heuristic_adslabs_NNH = AdsorbateSlabConfig(slab[0], adsorbate_NNH, mode="heuristic")
#Run relaxations
os.makedirs(f"data/{bulk_src_id}_H", exist_ok=True)
os.makedirs(f"data/{bulk_src_id}_NNH", exist_ok=True)
print(f'{len(heuristic_adslabs_H.atoms_list)} H slabs to compute for {bulk_src_id}')
print(f'{len(heuristic_adslabs_NNH.atoms_list)} NNH slabs to compute for {bulk_src_id}')
# Set up the calculator, note we're doing just the first 4 configs to keep this fast for the online documentation!
for idx, adslab in enumerate(heuristic_adslabs_H.atoms_list[:4]):
t0 = time.time()
adslab.calc = calc
print(f'Running data/{bulk_src_id}_H/{idx}')
opt = BFGS(adslab, trajectory=f"data/{bulk_src_id}_H/{idx}.traj", logfile=f"data/{bulk_src_id}_H/{idx}.log")
opt.run(fmax=0.05, steps=20)
print(f' Elapsed time: {time.time() - t0:1.1f} seconds for data/{bulk_src_id}_H/{idx}')
# Set up the calculator, note we're doing just the first 4 configs to keep this fast for the online documentation!
for idx, adslab in enumerate(heuristic_adslabs_NNH.atoms_list[:4]):
t0 = time.time()
adslab.calc = calc
print(f'Running data/{bulk_src_id}_NNH/{idx}')
opt = BFGS(adslab, trajectory=f"data/{bulk_src_id}_NNH/{idx}.traj", logfile=f"data/{bulk_src_id}_NNH/{idx}.log")
opt.run(fmax=0.05, steps=50)
print(f' Elapsed time: {time.time() - t0:1.1f} seconds for data/{bulk_src_id}_NNH/{idx}')
print(f'Elapsed time: {time.time() - tinit:1.1f} seconds')
0%| | 0/1 [00:00<?, ?it/s]
9 H slabs to compute for oqmd-349719
9 NNH slabs to compute for oqmd-349719
Running data/oqmd-349719_H/0
Elapsed time: 27.1 seconds for data/oqmd-349719_H/0
Running data/oqmd-349719_H/1
Elapsed time: 40.1 seconds for data/oqmd-349719_H/1
Running data/oqmd-349719_H/2
Elapsed time: 18.6 seconds for data/oqmd-349719_H/2
Running data/oqmd-349719_H/3
Elapsed time: 55.9 seconds for data/oqmd-349719_H/3
Running data/oqmd-349719_NNH/0
Elapsed time: 137.8 seconds for data/oqmd-349719_NNH/0
Running data/oqmd-349719_NNH/1
Elapsed time: 137.7 seconds for data/oqmd-349719_NNH/1
Running data/oqmd-349719_NNH/2
Elapsed time: 136.6 seconds for data/oqmd-349719_NNH/2
Running data/oqmd-349719_NNH/3
100%|██████████| 1/1 [11:30<00:00, 690.80s/it]
100%|██████████| 1/1 [11:30<00:00, 690.80s/it]
Elapsed time: 135.8 seconds for data/oqmd-349719_NNH/3
Elapsed time: 690.8 seconds
This cell runs all the examples. I don’t recommend you run this during the workshop. Instead, we have saved the results for the subsequent analyses so you can skip this one.
import time
from tqdm import tqdm
tinit = time.time()
for bulk_src_id in tqdm(bulk_ids):
# Enumerate slabs and establish adsorbates
bulk = Bulk(bulk_src_id_from_db=bulk_src_id, bulk_db_path="NRR_example_bulks.pkl")
slab = Slab.from_bulk_get_specific_millers(bulk= bulk, specific_millers=(1, 1, 1))
# Perform heuristic placements
heuristic_adslabs_H = AdsorbateSlabConfig(slab[0], adsorbate_H, mode="heuristic")
heuristic_adslabs_NNH = AdsorbateSlabConfig(slab[0], adsorbate_NNH, mode="heuristic")
#Run relaxations
os.makedirs(f"data/{bulk_src_id}_H", exist_ok=True)
os.makedirs(f"data/{bulk_src_id}_NNH", exist_ok=True)
print(f'{len(heuristic_adslabs_H.atoms_list)} H slabs to compute for {bulk_src_id}')
print(f'{len(heuristic_adslabs_NNH.atoms_list)} NNH slabs to compute for {bulk_src_id}')
# Set up the calculator
for idx, adslab in enumerate(heuristic_adslabs_H.atoms_list):
t0 = time.time()
adslab.calc = calc
print(f'Running data/{bulk_src_id}_H/{idx}')
opt = BFGS(adslab, trajectory=f"data/{bulk_src_id}_H/{idx}.traj", logfile=f"data/{bulk_src_id}_H/{idx}.log")
opt.run(fmax=0.05, steps=20)
print(f' Elapsed time: {time.time() - t0:1.1f} seconds for data/{bulk_src_id}_H/{idx}')
for idx, adslab in enumerate(heuristic_adslabs_NNH.atoms_list):
t0 = time.time()
adslab.calc = calc
print(f'Running data/{bulk_src_id}_NNH/{idx}')
opt = BFGS(adslab, trajectory=f"data/{bulk_src_id}_NNH/{idx}.traj", logfile=f"data/{bulk_src_id}_NNH/{idx}.log")
opt.run(fmax=0.05, steps=50)
print(f' Elapsed time: {time.time() - t0:1.1f} seconds for data/{bulk_src_id}_NNH/{idx}')
print(f'Elapsed time: {time.time() - tinit:1.1f} seconds')
Parse the trajectories and post-process#
As a post-processing step we check to see if:
the adsorbate desorbed
the adsorbate disassociated
the adsorbate intercalated
the surface has changed
We check these because they affect our referencing scheme and may result in energies that don’t mean what we think, e.g. they aren’t just adsorption, but include contributions from other things like desorption, dissociation or reconstruction. For (4), the relaxed surface should really be supplied as well. It will be necessary when correcting the SP / RX energies later. Since we don’t have it here, we will ommit supplying it, and the detector will instead compare the initial and final slab from the adsorbate-slab relaxation trajectory. If a relaxed slab is provided, the detector will compare it and the slab after the adsorbate-slab relaxation. The latter is more correct!
In this loop we find the most stable (most negative) adsorption energy for each adsorbate on each surface and save them in a DataFrame.
# Iterate over trajs to extract results
min_E = []
for file_outer in glob("data/*"):
ads = file_outer.split("_")[1]
bulk = file_outer.split("/")[1].split("_")[0]
results = []
for file in glob(f"{file_outer}/*.traj"):
rx_id = file.split("/")[-1].split(".")[0]
traj = ase.io.read(file, ":")
# Check to see if the trajectory is anomolous
detector = DetectTrajAnomaly(traj[0], traj[-1], traj[0].get_tags())
anom = (
detector.is_adsorbate_dissociated()
or detector.is_adsorbate_desorbed()
or detector.has_surface_changed()
or detector.is_adsorbate_intercalated()
)
rx_energy = traj[-1].get_potential_energy()
results.append({"relaxation_idx": rx_id, "relaxed_atoms": traj[-1],
"relaxed_energy_ml": rx_energy, "anomolous": anom})
df = pd.DataFrame(results)
df = df[~df.anomolous].copy().reset_index()
min_e = min(df.relaxed_energy_ml.tolist())
min_E.append({"adsorbate":ads, "bulk_id":bulk, "min_E_ml": min_e})
df = pd.DataFrame(min_E)
df_h = df[df.adsorbate == "H"]
df_nnh = df[df.adsorbate == "NNH"]
df_flat = df_h.merge(df_nnh, on = "bulk_id")
Make parity plots for values obtained by ML v. reported in the paper#
# Add literature data to the dataframe
with open("literature_data.pkl", "rb") as f:
literature_data = pickle.load(f)
df_all = df_flat.merge(pd.DataFrame(literature_data), on = "bulk_id")
f, (ax1, ax2) = plt.subplots(1, 2, sharey=True)
f.set_figheight(15)
x = df_all.min_E_ml_x.tolist()
y = df_all.E_lit_H.tolist()
ax1.set_title("*H parity")
ax1.plot([-3.5, 2], [-3.5, 2], "k-", linewidth=3)
slope, intercept, r, p, se = linregress(x, y)
ax1.plot(
[-3.5, 2],
[
-3.5 * slope + intercept,
2 * slope + intercept,
],
"k--",
linewidth=2,
)
ax1.legend(
[
"y = x",
f"y = {slope:1.2f} x + {intercept:1.2f}, R-sq = {r**2:1.2f}",
],
loc="upper left",
)
ax1.scatter(x, y)
ax1.axis("square")
ax1.set_xlim([-3.5, 2])
ax1.set_ylim([-3.5, 2])
ax1.set_xlabel("dE predicted OCP [eV]")
ax1.set_ylabel("dE NRR paper [eV]");
x = df_all.min_E_ml_y.tolist()
y = df_all.E_lit_NNH.tolist()
ax2.set_title("*N*NH parity")
ax2.plot([-3.5, 2], [-3.5, 2], "k-", linewidth=3)
slope, intercept, r, p, se = linregress(x, y)
ax2.plot(
[-3.5, 2],
[
-3.5 * slope + intercept,
2 * slope + intercept,
],
"k--",
linewidth=2,
)
ax2.legend(
[
"y = x",
f"y = {slope:1.2f} x + {intercept:1.2f}, R-sq = {r**2:1.2f}",
],
loc="upper left",
)
ax2.scatter(x, y)
ax2.axis("square")
ax2.set_xlim([-3.5, 2])
ax2.set_ylim([-3.5, 2])
ax2.set_xlabel("dE predicted OCP [eV]")
ax2.set_ylabel("dE NRR paper [eV]");
f.set_figwidth(15)
f.set_figheight(7)
/opt/hostedtoolcache/Python/3.12.9/x64/lib/python3.12/site-packages/scipy/stats/_stats_py.py:10730: RuntimeWarning: invalid value encountered in scalar divide
slope = ssxym / ssxm
/opt/hostedtoolcache/Python/3.12.9/x64/lib/python3.12/site-packages/scipy/stats/_stats_py.py:10744: RuntimeWarning: invalid value encountered in sqrt
t = r * np.sqrt(df / ((1.0 - r + TINY)*(1.0 + r + TINY)))
/opt/hostedtoolcache/Python/3.12.9/x64/lib/python3.12/site-packages/scipy/stats/_stats_py.py:10750: RuntimeWarning: invalid value encountered in scalar divide
slope_stderr = np.sqrt((1 - r**2) * ssym / ssxm / df)
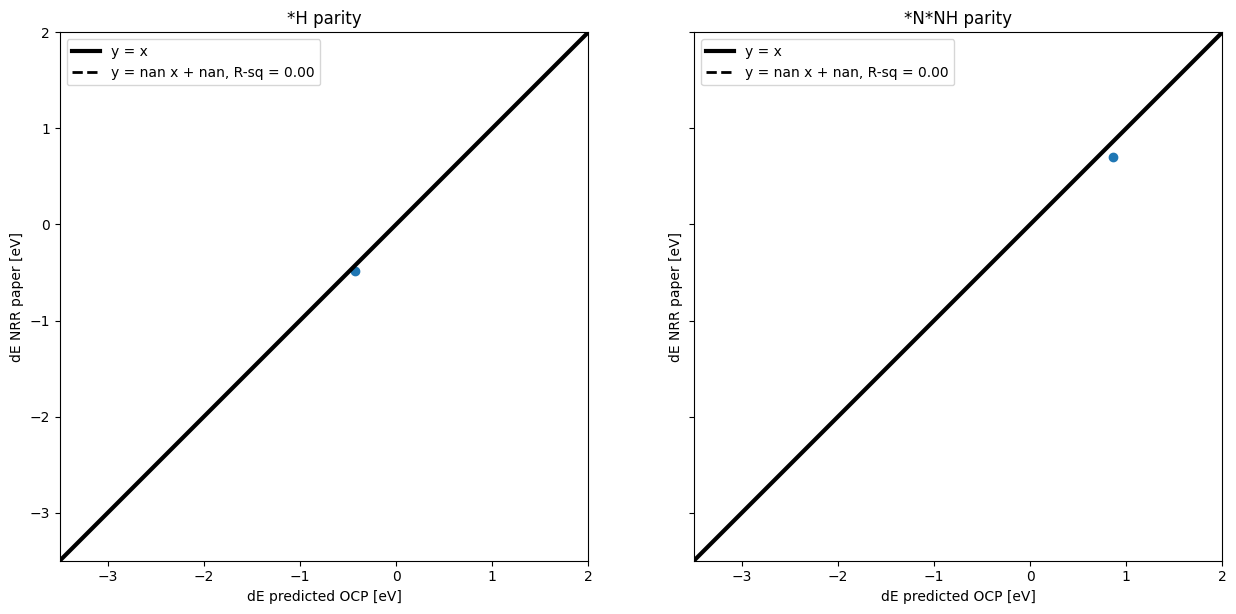
Make figure 6b and compare to literature results#
f, (ax1, ax2) = plt.subplots(1, 2, sharey=True)
x = df_all[df_all.reaction == "HER"].min_E_ml_y.tolist()
y = df_all[df_all.reaction == "HER"].min_E_ml_x.tolist()
comp = df_all[df_all.reaction == "HER"].composition.tolist()
ax1.scatter(x, y,c= "r", label = "HER")
for i, txt in enumerate(comp):
ax1.annotate(txt, (x[i], y[i]))
x = df_all[df_all.reaction == "NRR"].min_E_ml_y.tolist()
y = df_all[df_all.reaction == "NRR"].min_E_ml_x.tolist()
comp = df_all[df_all.reaction == "NRR"].composition.tolist()
ax1.scatter(x, y,c= "b", label = "NRR")
for i, txt in enumerate(comp):
ax1.annotate(txt, (x[i], y[i]))
ax1.legend()
ax1.set_xlabel("dE *N*NH predicted OCP [eV]")
ax1.set_ylabel("dE *H predicted OCP [eV]")
x = df_all[df_all.reaction == "HER"].E_lit_NNH.tolist()
y = df_all[df_all.reaction == "HER"].E_lit_H.tolist()
comp = df_all[df_all.reaction == "HER"].composition.tolist()
ax2.scatter(x, y,c= "r", label = "HER")
for i, txt in enumerate(comp):
ax2.annotate(txt, (x[i], y[i]))
x = df_all[df_all.reaction == "NRR"].E_lit_NNH.tolist()
y = df_all[df_all.reaction == "NRR"].E_lit_H.tolist()
comp = df_all[df_all.reaction == "NRR"].composition.tolist()
ax2.scatter(x, y,c= "b", label = "NRR")
for i, txt in enumerate(comp):
ax2.annotate(txt, (x[i], y[i]))
ax2.legend()
ax2.set_xlabel("dE *N*NH literature [eV]")
ax2.set_ylabel("dE *H literature [eV]")
f.set_figwidth(15)
f.set_figheight(7)
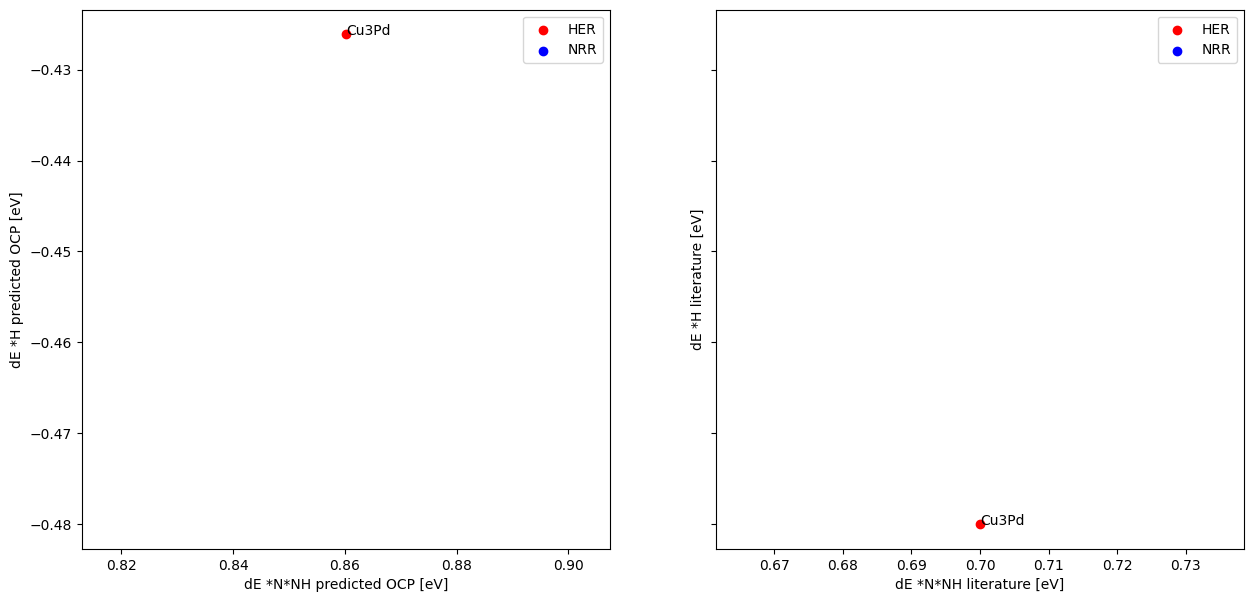